Machine learning techniques have demonstrated super-human abilities that are necessary towards improving the recycling infrastructure. Many waste objects come to plants deformed, and as models are trained on perfect data, they exhibit poor accuracy when it comes to real waste analysis. The research carried out provides an approach that can generate an unlimited amount of synthetic deformed data. This approach approximates complex geometry with a bounding cylinder, mapping deformation point clouds back to the host model to curate probable deformed objects without the need for complex analysis or generative networks.
Deformation Simulations
Using an open-source 3D modelling software, rigging, animation, simulations, rendering, compositing and motion tracking of objects could be achieved. Objects can be made to collide on this software to generate probable crushed representations.
The Cylindrical Shell
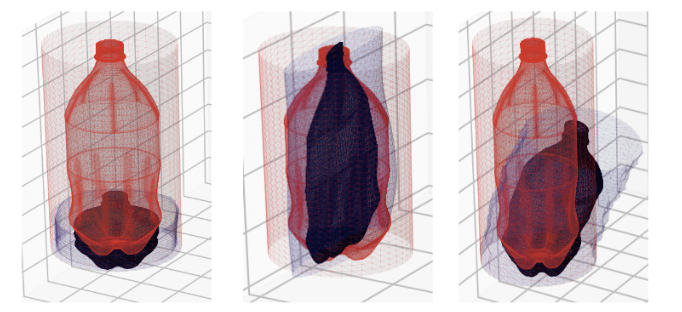
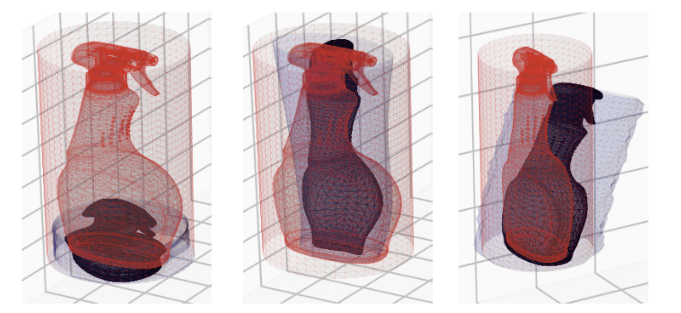
Using a k-nearest-neighbour matching deformation consolidation algorithm, an inverse cube function, and sufficient model granularity, a cylindrical deformation cloud was projected onto an original object shape to showcase what might have happened if it were loaded differently. Prior object mesh (red) and prior approximated cylinder (red), along with the deformed cylinder (blue) and post object mesh (black).
A Structural Solution
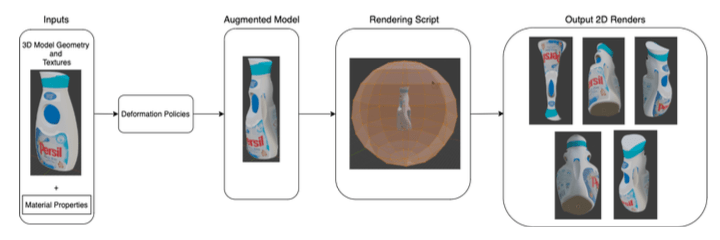
This image above visualises a high-level 3D augmentation and deformation pipeline.
Experimental Results

These images showcase the real carton on the left and synthetic carton on the right, visualising it with no deformations and with deformations from the test dataset.
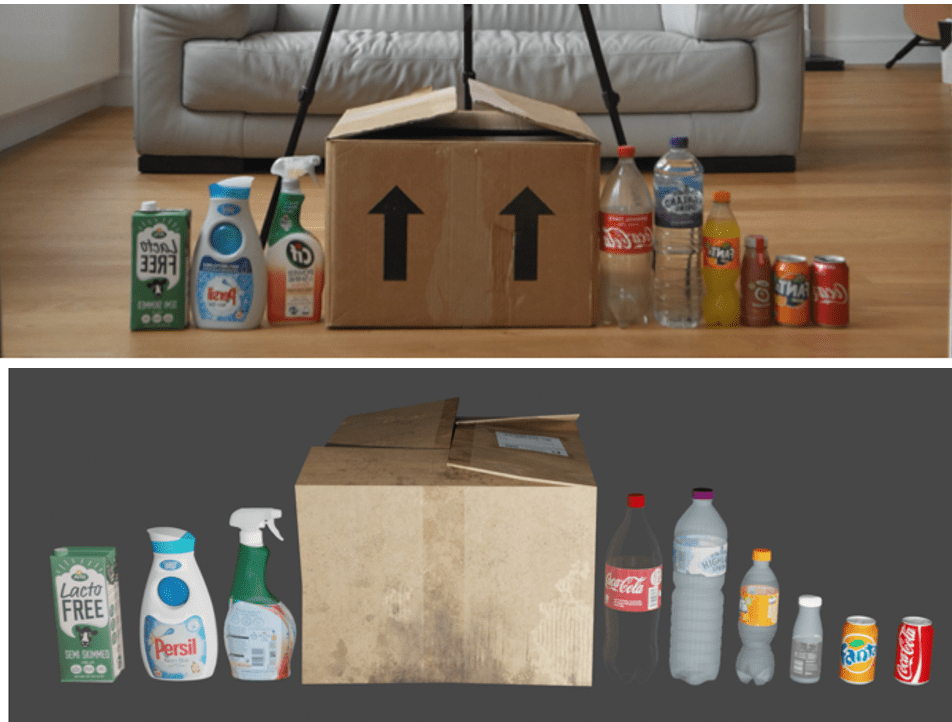
Showcased is a sample of the physical packaging classes used to generate the dataset (top) and all digital 3D models of the objects used to create augmented and non-augmented renders for training purposes.
In Conclusion
Ground-breaking research was achieved to produce valuable datasets, and the resulting deformed, synthetic training data led to an improved network performance in comparison to non-deformed data for a fixed architecture and training regime.