The advancements in deep learning have enabled rapid growth of computer vision capabilities. However, in order to achieve successful results, it requires a heavy reliance on the availability of large labelled datasets. This proves to be problematic for tasks such as instance segmentation, which entails many real-life applications. Moreover, datasets of the required scale with relevant annotations often do not exist, and obtaining precise pixel-level masks can be very expensive.
This research addresses the weaknesses of traditional deep learning methods on low labelled data regimes. This is done by exploring the limitations of transfer learning and proposing a novel semi-supervised learning approach for instance segmentation, which is based on self-training and implicit consistency regularisation through data augmentations. Most significantly, it is shown that even with access to a limited number of labelled images that cover a limited number of classes, a model’s ability to generalise to novel classes can show notable improvements through leveraging raw, unlabelled data.
Deformation Simulations
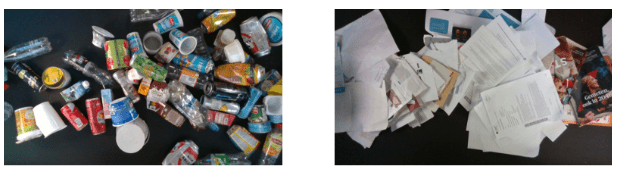
Training Set
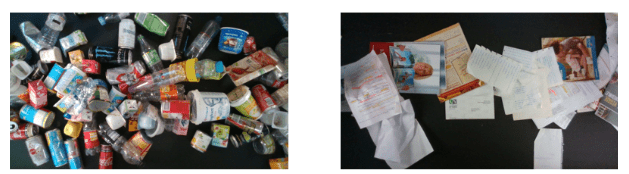
Validation set
The images above showcase: the training set and the validation/holdout set. Group 1, as shown on the left set of images, is comprised of waste items such as cans, yogurt cups, cylindrical tins, Tetra Paks, and PET bottles. Group 2, as shown on the right set of images, includes paper and magazine waste materials.
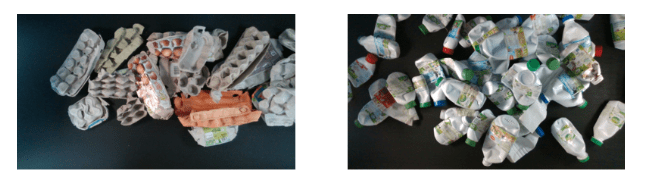
Egg cartons EGGC (left), HDPE milk cartons (right)
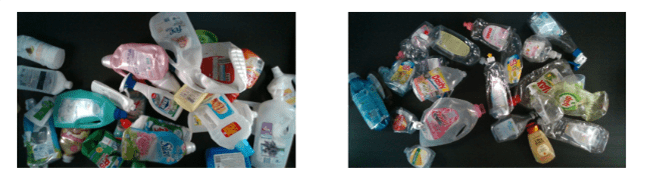
Non-food grade opaque plastic NFGO (left), Non-food grade transparent plastic NFGT (right)
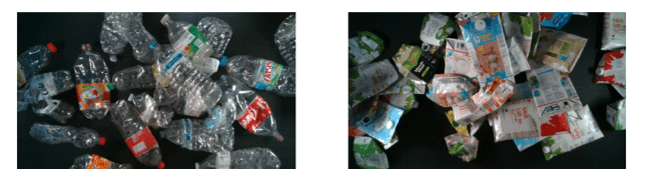
Large PET bottles PETL (left), Large tetra paks TETL (right)
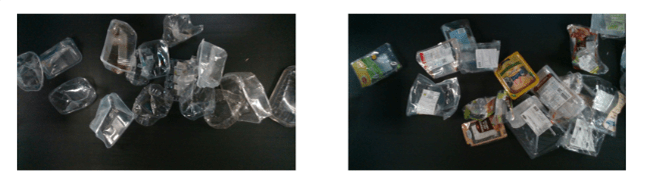
Deep transparent plastic trays TRAD (left), flat transparent plastic trays TRAF (right)
These images showcase those used as the test set representing the novel classes
Experimentation

Cans and bottles (Group 1)

Paper and magazines (Group 2)

Egg cartons (EGGC)

HDPE milk cartons (HDPE)

Non-food grade transparent plastics (NFGT)
Images from the holdout set – a representative example selected from each group, and test set that shows ground truth masks on the left, predicted masks with baseline model in the middle, and predicted masks with the same model using the semi-supervised learning approach on the right.
In Conclusion
The results show that it is possible to obtain relatively high quality results even when labelled data is limited. This was concluded with an appropriate implementation of transfer learning methods, using state-of-the-art instance segmentation models equipped with sufficiently powerful encoder backbones, and an effective data augmentation strategy.